Cockpit AI Agent: Autonomous scenario creation becomes the first step to personalize cockpits
In AI Foundation Models’ Impacts on Vehicle Intelligent Design and Development Research Report, 2024, ResearchInChina mentioned that the core of an AI Agent uses a large language model (LLM) as its core computing engine (LLM OS). In the AI service framework, the LLM acts as AI core and the Agent acts as AI APP.? With the help of reasoning and generation capabilities of? AI foundation model, the Agent can create more cockpit scenarios, while further improving current multimodal interaction, voice processing and other technologies in the cockpit.
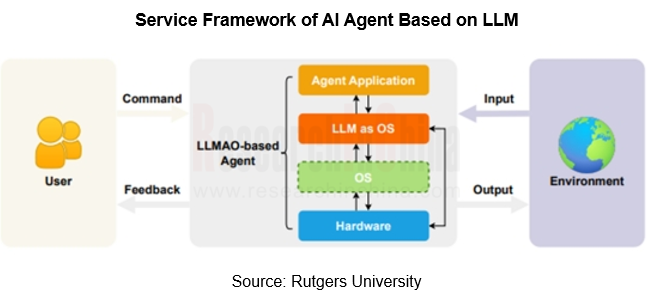
Compared with the traditional OS, AI OS is programmed in natural language, which is more suitable for human operation and convenient for customization and personalized human-computer interaction. The Agent responds to user prompts or instructions in natural language, and can perform a lot of tasks according to its interaction with physical or digital environment.
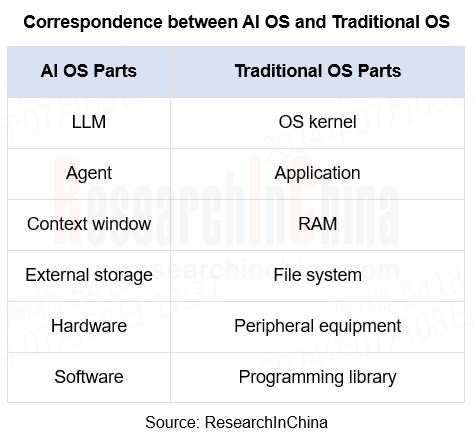
Foundation models spawn Agent customized scenarios
Since AI foundation models were installed in cars, they have been widely used in multimodal interaction scenarios. OEMs have started from voice assistants to further improve? intelligent processing of user commands:
Before foundation models got on cars, the wake-up rate and effective instructions of voice assistants in cockpits are at a low level;
After foundation models were available in cars, not only has voice recognition become more intelligent, but the application of expression monitoring and gesture control have also been improved, with effective commands and daily active rates increasing significantly.
AI foundation models further enhance the user experience of smart cockpits through strong context processing and reasoning capabilities. Please refer to the following dialogue:
(Mobility scenario)
--**Tongxue! (the name of a voice assistant)
-I am here!
-There seems to be a prairie ahead. Please take a picture for me!
-Wait a moment, and I will capture the beautiful scenariory of the prairie for you. I'll let you know when the photo is taken. Is there anything else I can help you with?
-Send it to Moments immediately. I want my friends to have a look at this beautiful scenariory.
-Ok, according to your authorized account, please choose the material and filter style.
-Just this one, with a caption.
-Ok, three captions have been generated for you. Which one do you like?
-Just this one.
(You enter the office and receive a phone call)
-There is a phone call from a customer, and I have transferred it to the IVI for you.? Would you like to answer it?
-Yes, and turn on the office mode.
-Ok, I will generate an avatar of the customer for you and start the conversation. Do you have any questions or things to discuss? I will record the conversation and provide you with the minutes of the meeting after it is over.
(The avatar is generated)
-Now you can start the conversation.
The above scenarios will not be materialized in the cockpit until 2024 when foundation models are installed on vehicles by some OEMs.
For example, IM L6 has built Carlog and City Drive scenarios to enable the AI foundation models to proactively recommend food and attractions and allow users to post them on social media:
Carlog: Actively perceive the scenario during driving through AI vision foundation model, mobilize four cameras to take photos, automatically save and edit them, and support one-click share in Moments.
City Drive: Cooperate with Volcengine to model nearby food, scenic spots and landmarks in real time in the digital screen, and push them according to users' habits and preferences.
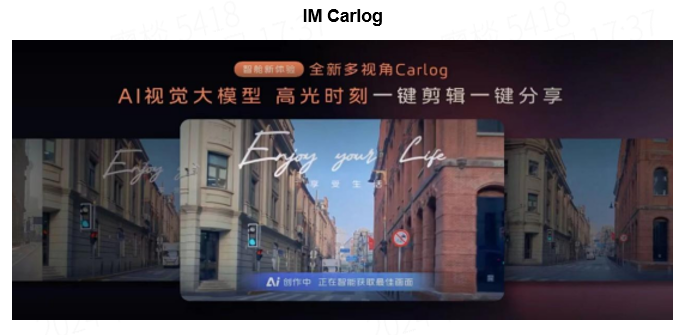
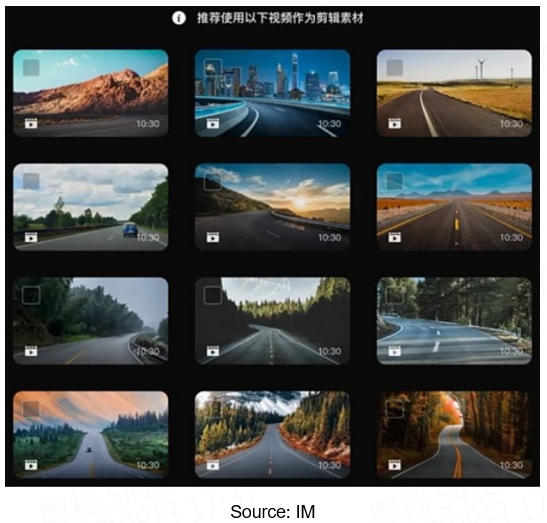
The applicability of foundation models in various scenarios has stimulated users' demand for intelligent agents that can uniformly manage cockpit functions. In 2024, OEMs such as NIO, Li Auto, and Hozon successively launched Agent frameworks, using voice assistants as the starting point to manage functions and applications in cockpits.
Agent service frameworks can not only manage cockpit functions in a unified way, but also provide more abundant scenario modes according to customers' needs and preferences, especially supporting customized scenarios for users, which accelerates the advent of the cockpit personalization era.
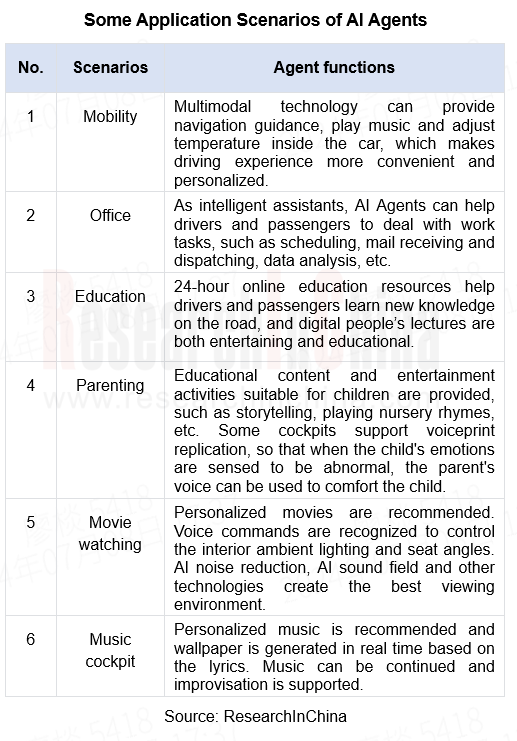
For example, NIO’s NOMI GPT allows users to set an AI scenario with just one sentence:
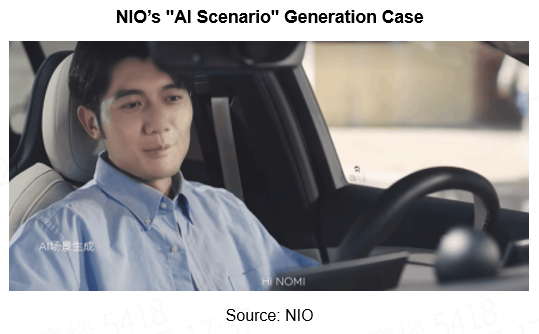
Core competence of cockpit Agents
AI Agents in the era of foundation models are based on LLMs, whose powerful reasoning expands the applicable scenarios of AI Agents that can improve the thinking capability of foundation models through feedback obtained during operation. In the cockpit, the Agent capability paradigm can be roughly divided into "Understanding" + "Planning" + "Tool Use" + "Reflection".
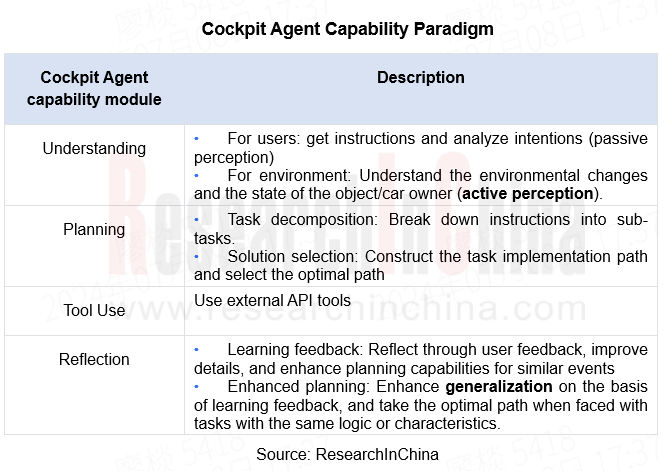
When Agents first get on cars, cognitive and planning abilities are more important. The understanding of task goals and the choice of implementation paths directly determine the accuracy of performance results, which in turn affect the scenario utilization rate of Agents.
For example, in Xiaomi's voice interaction process, semantic understanding is the difficulty of the entire automotive voice processing process. XiaoAi handles semantic parsing through a semantic parsing model.
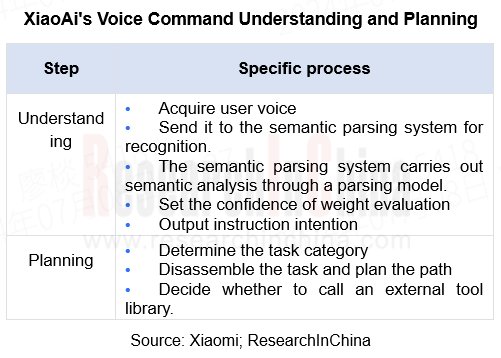
After the mass production of Agents, the personalized cockpits that support users to customize scenario modes become the highlight, and Reflection becomes the most important core competence at this stage, so it is necessary to build an Agentic Workflow that is constantly learning and optimizing.
For example, Lixiang Tongxue offered by Li Auto supports the creation of one-sentence scenarios. It is backed by Mind GPT's built-in memory network and online reinforcement learning capabilities. Mind GPT can remember personalized preferences and habits based on historical conversations. When similar scenarios recur, it can automatically set scenario parameters through historical data to fit the user's original intentions.
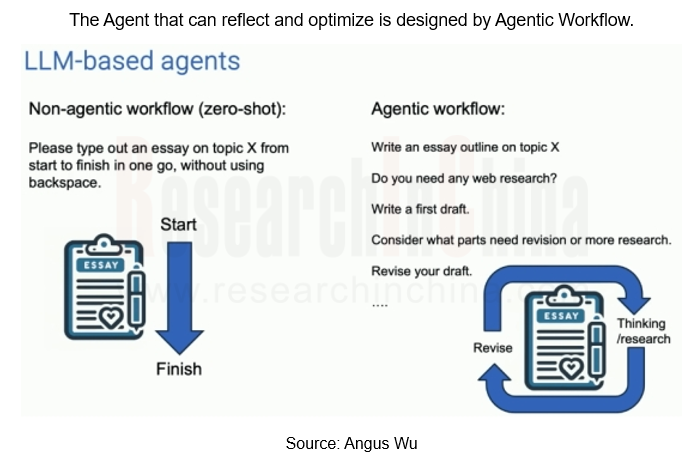
At the AI OS architecture setting level, we take SAIC Z-One as an example:
Z-One accesses the LLM kernel (LLM OS) at the kernel layer, which controls the interfaces of AI OS SDK and ASF with the original microkernel respectively, in which AI OS SDK receives the scheduling of the LLM to promote the Agent service framework of the application layer. The Z-One AI OS architecture highly integrates AI with CPU. Through SOA atomic services, AI is then connected to the vehicle's sensors, actuators and controllers. This architecture, based on a terminal-cloud foundation model, can enhance the computing power of the terminal-side foundation model and reduce operational latency.
Application Difficulty of Cockpit AI Agents
Agents connect to users and execute commands. In the application process, in addition to the technical difficulties of putting foundation models on cars, they also face scenario difficulties. In the process of command reception-semantic analysis-intention reasoning-task execution, the accuracy of the performance results and the delay in human-computer interaction directly affect the user's riding experience.
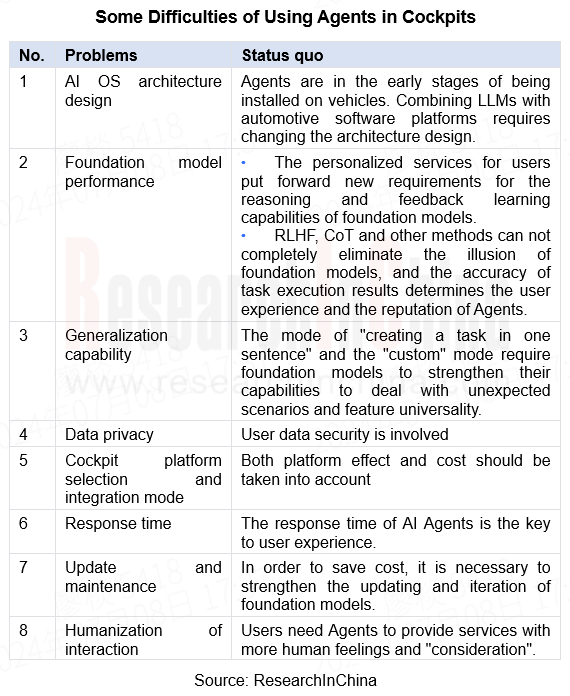
Humanization of interaction
For example, in the "emotional consultant" scenario, Agents should resonate emotionally with car owners and perform anthropomorphism. Generally, there are three forms of anthropomorphism of AI Agents: physical anthropomorphism, personality anthropomorphism, and emotional anthropomorphism.
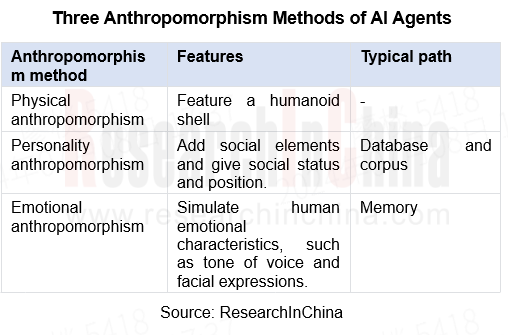
NIO's NOMI GPT uses "personality anthropomorphism" and "emotional anthropomorphism":
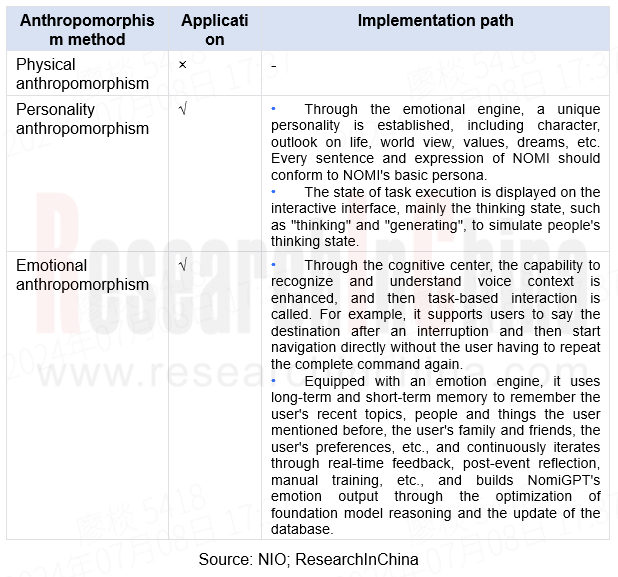
Foundation model performance
In the "encyclopedia question and answer" scenario, Agents may be unable to answer the user's questions, especially open questions, accurately because of LLM illusion after semantic analysis, database search, answer generation and the like.
Current solutions include advanced prompting, RAG+knowledge graph, ReAct, CoT/ToT, etc., which cannot completely eliminate “LLM illusion”. In the cockpit, external databases, RAG, self-consistency and other methods are more often used to reduce the frequency of “LLM illusion”.
Some foundation model manufacturers have improved the above solutions. For example, Meta has proposed to reduce “LLM illusion” through Chain-of-Verification (CoVe). This method breaks down fact-checking into more detailed sub-questions to improve response accuracy and is consistent with the human-driven fact-checking process. It can effectively improve the FACTSCORE indicator in long-form generation tasks.
CoVe includes four steps: query, plan verification, execute verification and? final verified response.
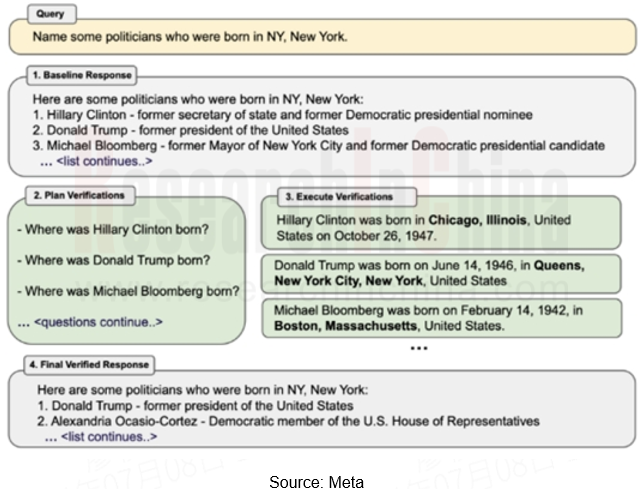
Automotive MEMS (Micro Electromechanical System) Sensor Research Report, 2025
Automotive MEMS Research: A single vehicle packs 100+ MEMS sensors, and the pace of product innovation and localization are becoming much faster.
MEMS (Micro Electromechanical System) is a micro devi...
Intelligent Vehicle Cockpit-driving Integration (Cockpit-driving-parking) Industry Report, 2024-2025
Cockpit-driving integration is gaining momentum, and single-chip solutions are on the horizon
The Intelligent Vehicle Cockpit-driving Integration (Cockpit-driving-parking) Industry Repor...
Automotive TSP and Application Service Research Report, 2024-2025
TSP Research: In-vehicle connectivity services expand in the direction of cross-domain integration, all-scenario integration and cockpit-driving integration
TSP (Telematics Service Provider) is mainl...
Autonomous Driving Domain Controller and Central Control Unit (CCU) Industry Report, 2024-2025
Autonomous Driving Domain Controller Research: One Board/One Chip Solution Will Have Profound Impacts on the Automotive Supply Chain
Three development stages of autonomous driving domain controller:...
Global and China Range Extended Electric Vehicle (REEV) and Plug-in Hybrid Electric Vehicle (PHEV) Research Report, 2024-2025
Research on REEV and PHEV: Head in the direction of high thermal efficiency and large batteries, and there is huge potential for REEVs to go overseas
In 2024, hybrid vehicles grew faster than batter...
Automotive AI Agent Product Development and Commercialization Research Report, 2024
Automotive AI Agent product development: How to enable “cockpit endorser” via foundation models?
According to OPEN AI’s taxonomy of AI (a total of 5 levels), AI Agent is at L3 in the AI development ...
China ADAS Redundant System Strategy Research Report, 2024
Redundant system strategy research: develop towards integrated redundant designADAS redundant system definition framework
For autonomous vehicles, safety is the primary premise. Only when ADAS is ful...
Smart Car OTA Industry Report, 2024-2025
Automotive OTA research: With the arrival of the national mandatory OTA standards, OEMs are accelerating their pace in compliance and full life cycle operations
The rising OTA installations facilitat...
End-to-end Autonomous Driving Industry Report, 2024-2025
End-to-end intelligent driving research: How Li Auto becomes a leader from an intelligent driving follower
There are two types of end-to-end autonomous driving: global (one-stage) and segmented (two-...
China Smart Door and Electric Tailgate Market Research Report, 2024
Smart door research: The market is worth nearly RMB50 billion in 2024, with diverse door opening technologies
This report analyzes and studies the installation, market size, competitive landsc...
Commercial Vehicle Intelligent Chassis Industry Report, 2024
Commercial vehicle intelligent chassis research: 20+ OEMs deploy chassis-by-wire, and electromechanical brake (EMB) policies are expected to be implemented in 2025-2026
The Commercial Vehicle Intell...
Automotive Smart Surface Industry Report, 2024
Research on automotive smart surface: "Plastic material + touch solution" has become mainstream, and sales of smart surface models soared by 105.1% year on year
In this report, smart surface refers t...
China Automotive Multimodal Interaction Development Research Report, 2024
Multimodal interaction research: AI foundation models deeply integrate into the cockpit, helping perceptual intelligence evolve into cognitive intelligence
China Automotive Multimodal Interaction Dev...
Automotive Vision Industry Report, 2024
Automotive Vision Research: 90 million cameras are installed annually, and vision-only solutions lower the threshold for intelligent driving. The cameras installed in new vehicles in China will hit 90...
Automotive Millimeter-wave (MMW) Radar Industry Report, 2024
Radar research: the pace of mass-producing 4D imaging radars quickens, and the rise of domestic suppliers speeds up.
At present, high-level intelligent driving systems represented by urban NOA are fa...
Chinese Independent OEMs’ ADAS and Autonomous Driving Report, 2024
OEM ADAS research: adjust structure, integrate teams, and compete in D2D, all for a leadership in intelligent driving
In recent years, China's intelligent driving market has experienced escala...
Research Report on Overseas Layout of Chinese Passenger Car OEMs and Supply Chain Companies, 2024
Research on overseas layout of OEMs: There are sharp differences among regions. The average unit price of exports to Europe is 3.7 times that to Southeast Asia.
The Research Report on Overseas Layou...
In-vehicle Payment and ETC Market Research Report, 2024
Research on in-vehicle payment and ETC: analysis on three major application scenarios of in-vehicle payment
In-vehicle payment refers to users selecting and purchasing goods or services in the car an...