End-to-end Autonomous Driving Research: status quo of End-to-end (E2E) autonomous driving
1. Status quo of end-to-end solutions in China
An end-to-end autonomous driving system refers to direct mapping from sensor data inputs (camera images, LiDAR, etc.) to control command outputs (steering, acceleration/deceleration, etc.). It first appeared in the ALVINN project in 1988. It uses cameras and laser rangefinders as input and a simple neural network to generate steering as output.
In early 2024, Tesla rolled out FSD V12.3, featuring an amazing intelligent driving level. The end-to-end autonomous driving solution garners widespread attention from OEMs and autonomous driving solution companies in China.?
Compared with conventional multi-module solutions, the end-to-end autonomous driving solution integrates perception, prediction and planning into a single model, simplifying the solution structure. It can simulate human drivers making driving decisions directly according to visual inputs, effectively cope with long tail scenarios of modular solutions and improve the training efficiency and performance of models.
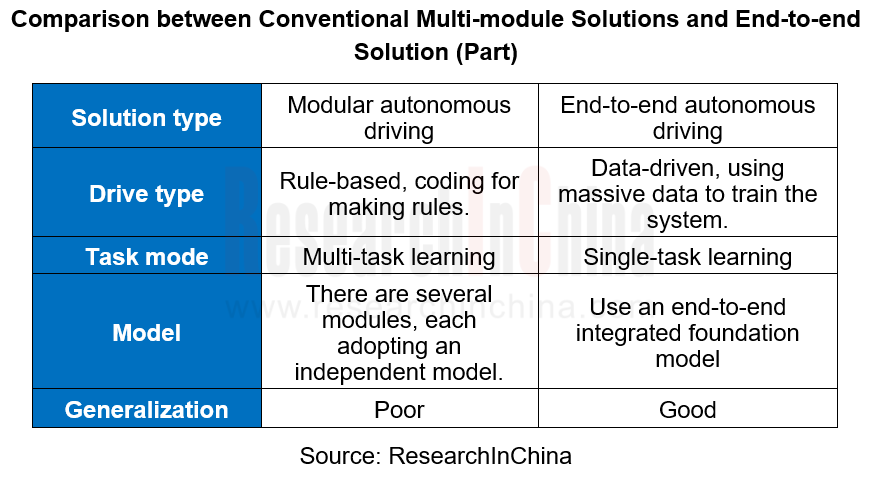
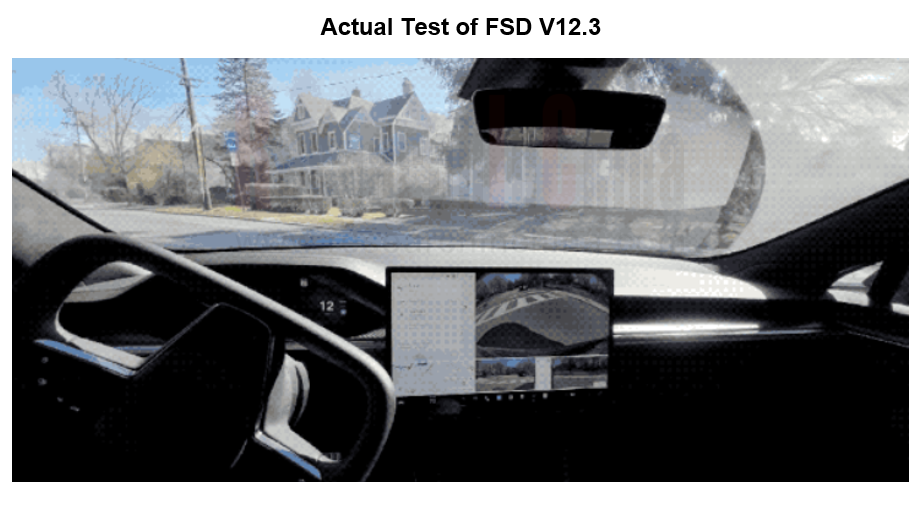
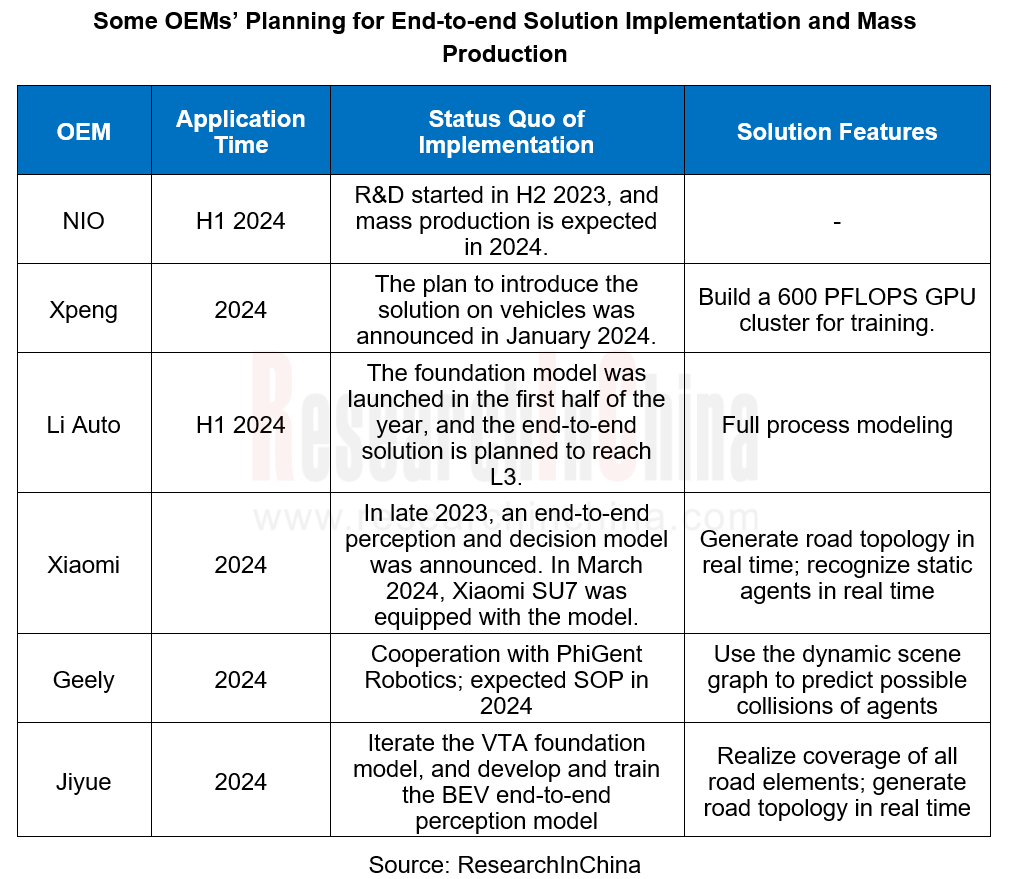
Li Auto's end-to-end solution
Li Auto believes that a complete end-to-end model should cover the whole process of perception, tracking, prediction, decision and planning, and it is the optimal solution to achieve L3 autonomous driving. In 2023, Li Auto pushed AD Max3.0, with overall framework reflecting the end-to-end concept but still a gap with a complete end-to-end solution. In 2024, Li Auto is expected to promote the system to become a complete end-to-end solution.?
Li Auto's autonomous driving framework is shown below, consisting of two systems:
Fast system: System 1, Li Auto’s existing end-to-end solution which is directly executed after perceiving the surroundings.
Slow system: System 2, a multimodal large language model that logically thinks and explores unknown environments to solve problems in unknown L4 scenarios.
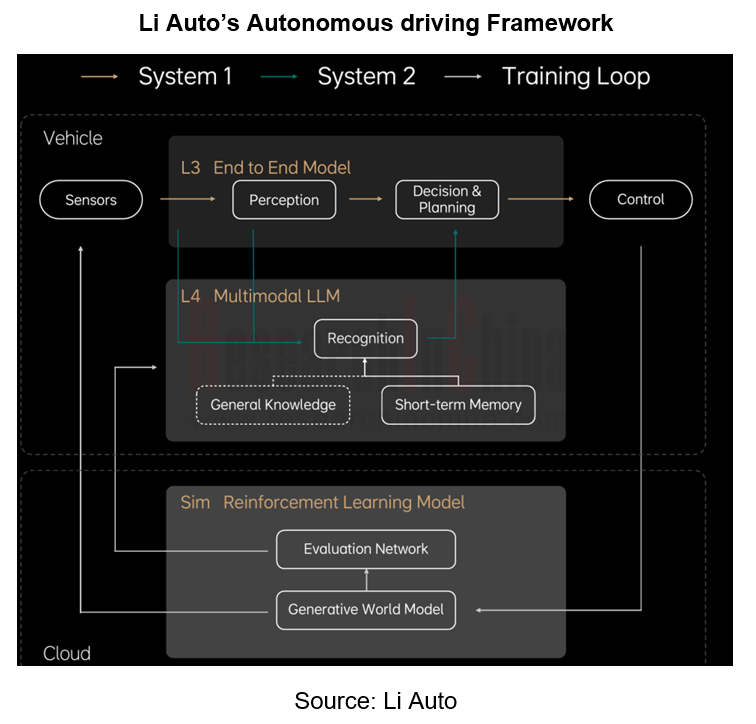
In the process of promoting the end-to-end solution, Li Auto plans to unify the planning/forecast model and the perception model, and accomplish the end-to-end Temporal Planner on the original basis to integrate parking with driving.
2. Data becomes the key to the implementation of end-to-end solutions.
The implementation of an end-to-end solution requires processes covering R&D team building, hardware facilities, data collection and processing, algorithm training and strategy customization, verification and evaluation, promotion and mass production. Some of the sore points in scenarios are as shown in the table:
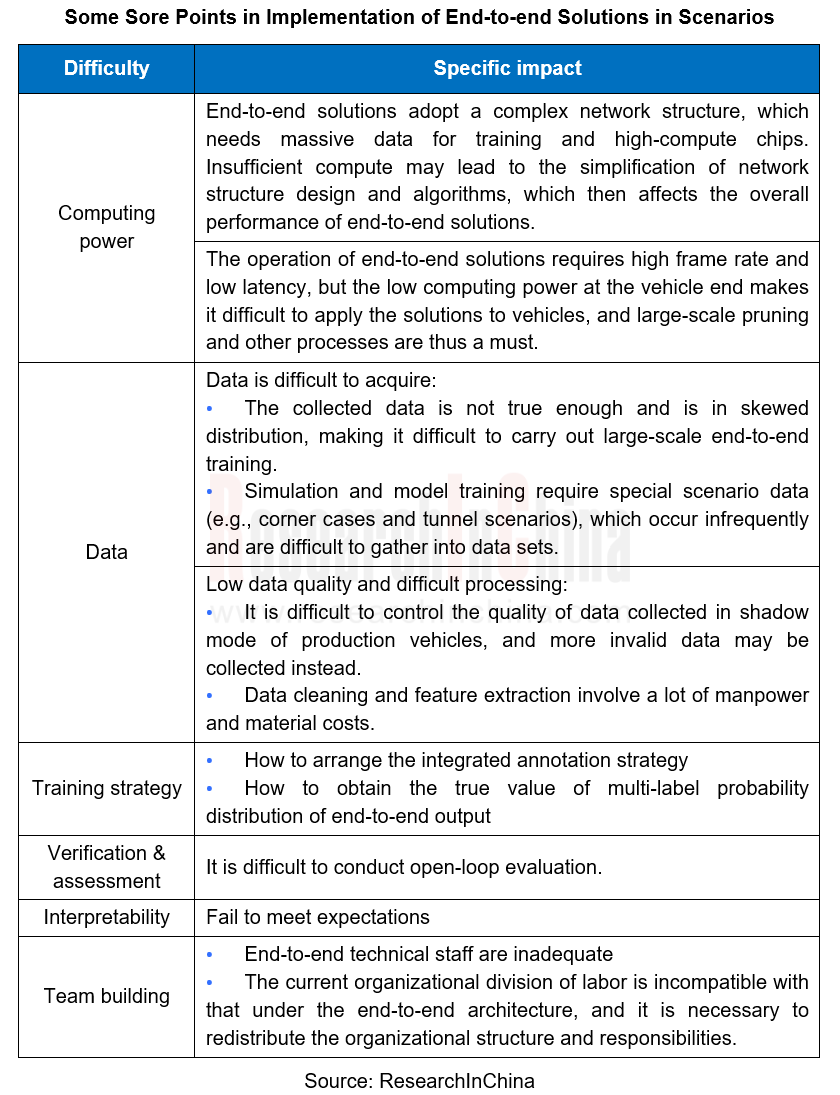
The integrated training in end-to-end autonomous driving solutions requires massive data, so one of the difficulties it faces lies in data collection and processing.
First of all, it needs a long time and may channels to collect data, including driving data and scenario data such as roads, weather and traffic conditions. In actual driving, the data within the driver's front view is relatively easy to collect, but the surrounding information is hard to say.
During data processing, it is necessary to design data extraction dimensions, extract effective features from massive video clips, make statistics of data distribution, etc. to support large-scale data training.
DeepRoute
As of March 2024, DeepRoute.ai's end-to-end autonomous driving solution has been designated by Great Wall Motor and involved in the cooperation with NVIDIA. It is expected to adapt to NVIDIA Thor in 2025. In the planning of DeepRoute.ai, the transition from the conventional solution to the "end-to-end" autonomous driving solution will go through sensor pre-fusion, HD map removal, and integration of perception, decision and control.
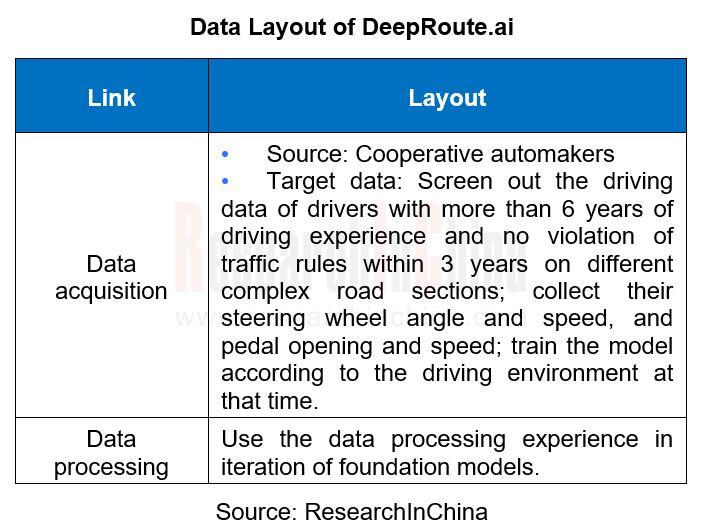
GigaStudio
DriveDreamer, an autonomous driving model of GigaStudio, is capable of scenario generation, data generation, driving action prediction and so forth. In the scenario/data generation, it has two steps:
When involving single-frame structural conditions, guide DriveDreamer to generate driving scenario images, so that it can understand structural traffic constraints easily.
Extend its understanding to video generation. Using continuous traffic structure conditions, DriveDreamer outputs driving scene videos to further enhance its understanding of motion transformation.
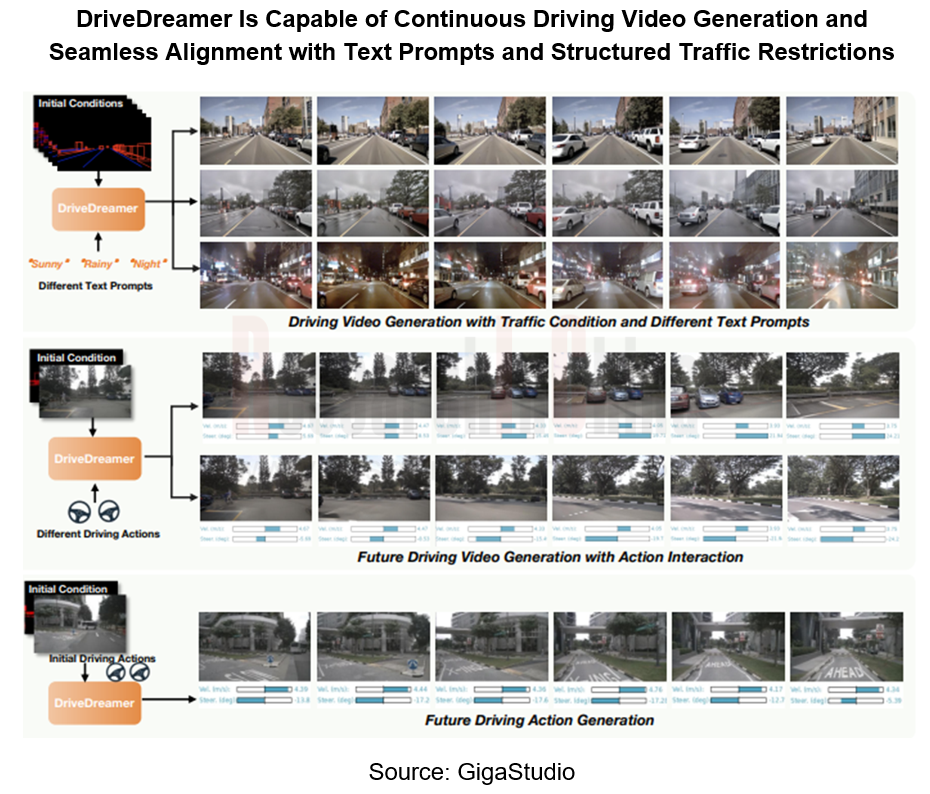
3. End-to-end solutions accelerate the application of embodied robots.
In addition to autonomous vehicles, embodied robots are another mainstream scenario of end-to-end solutions. From end-to-end autonomous driving to robots, it is necessary to build a more universal world model to adapt to more complex and diverse real application scenarios. The development framework of mainstream AGI (General Artificial Intelligence) is divided into two stages:
Stage 1: the understanding and generation of basic foundation models are unified, and further combined with embodied artificial intelligence (embodied AI) to form a unified world model;
Stage 2: capabilities of world model + complex task planning and control, and abstract concept induction gradually evolve into the era of the interactive AGI 1.0.
In the landing process of the world model, the construction of an end-to-end VLA (Vision-Language-Action) autonomous system has become a crucial link. VLA, as the basic foundation model of embodied AI, can seamlessly link 3D perception, reasoning and action to form a generative world model, which is built on the 3D-based large language model (LLM) and introduces a set of interactive markers to interact with the environment.
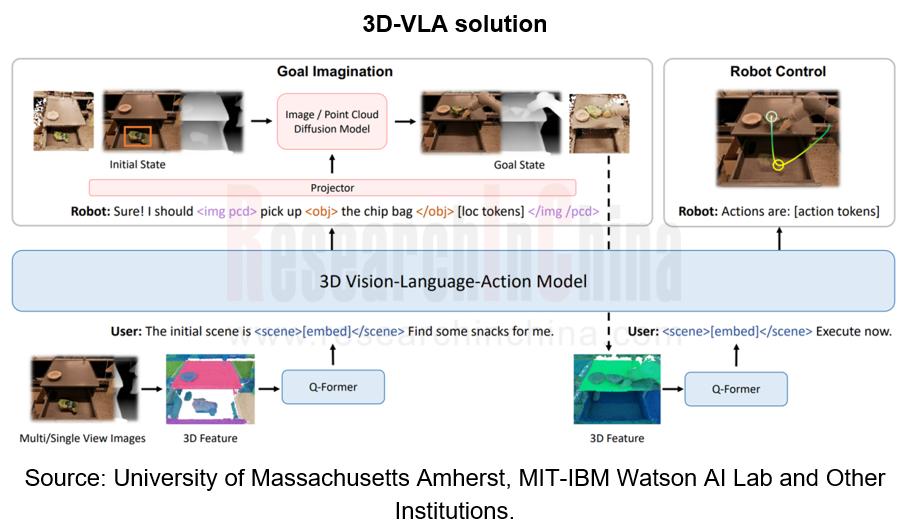
As of April 2024, some manufacturers of humanoid robots adopting end-to-end solutions are as follows:
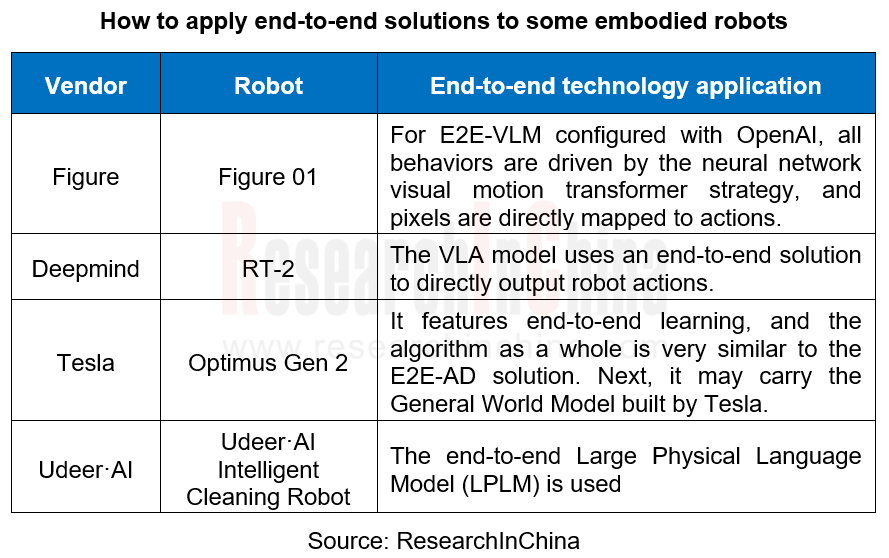
For example, Udeer·AI's Large Physical Language Model (LPLM) is an end-to-end embodied AI solution that uses a self-labeling mechanism to improve the learning efficiency and quality of the model from unlabeled data, thereby deepening the understanding of the world and enhancing the robot's generalization capabilities and environmental adaptability in cross-modal, cross-scene, and cross-industry scenarios.
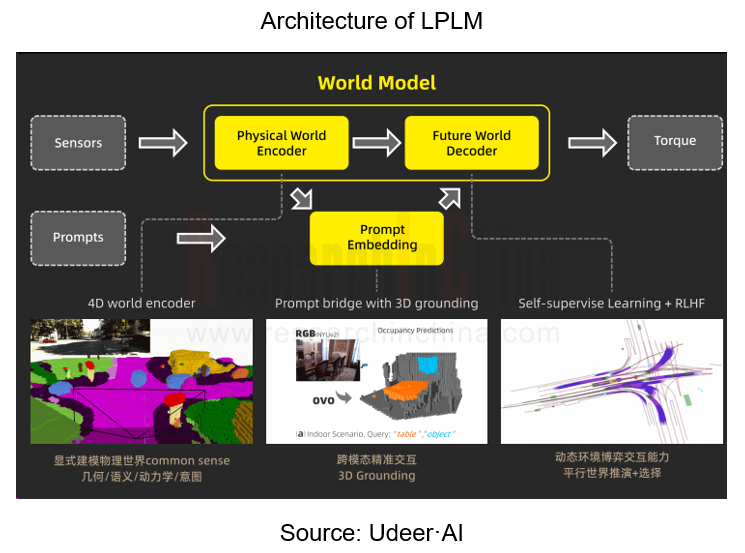
LPLM abstracts the physical world and ensures that this kind of information is aligned with the abstract level of features in LLM. It explicitly models each entity in the physical world as a token, and encodes geometric, semantic, kinematic and intentional information.
In addition, LPLM adds 3D grounding to the encoding of natural language instructions, improving the accuracy of natural language to some extent. Its decoder can learn by constantly predicting the future, thus strengthening the ability of the model to learn from massive unlabeled data.
Automotive Micromotor and Motion Mechanism Industry Report, 2025
Automotive Micromotor and Motion Mechanism Research: More automotive micromotors and motion mechanisms are used in a single vehicle, especially in cockpits, autonomous driving and other scenarios.
Au...
Research Report on AI Foundation Models and Their Applications in Automotive Field, 2024-2025
Research on AI foundation models and automotive applications: reasoning, cost reduction, and explainability
Reasoning capabilities drive up the performance of foundation models.
Since the second ha...
China's New Passenger Cars and Suppliers' Characteristics Research Report, 2024-2025
Trends of new cars and suppliers in 2024-2025: New in-vehicle displays are installed, promising trend of AI and cars is coming
ResearchInChina releases the China's New Passenger Cars and Suppli...
Global and China Skateboard Chassis Industry Report, 2024-2025
Skateboard chassis research: already used in 8 production models, and larger-scale production expected beyond 2025
Global and China Skateboard Chassis Industry Report, 2024-2025 released by ResearchI...
Two-wheeler Intelligence and Industry Chain Research Report, 2024-2025
Research on the two-wheeler intelligence: OEMs flock to enter the market, and the two-wheeler intelligence continues to improve
This report focuses on the upgrade of two-wheeler intelligence, analyz...
Automotive MEMS (Micro Electromechanical System) Sensor Research Report, 2025
Automotive MEMS Research: A single vehicle packs 100+ MEMS sensors, and the pace of product innovation and localization are becoming much faster.
MEMS (Micro Electromechanical System) is a micro devi...
Intelligent Vehicle Cockpit-driving Integration (Cockpit-driving-parking) Industry Report, 2024-2025
Cockpit-driving integration is gaining momentum, and single-chip solutions are on the horizon
The Intelligent Vehicle Cockpit-driving Integration (Cockpit-driving-parking) Industry Repor...
Automotive TSP and Application Service Research Report, 2024-2025
TSP Research: In-vehicle connectivity services expand in the direction of cross-domain integration, all-scenario integration and cockpit-driving integration
TSP (Telematics Service Provider) is mainl...
Autonomous Driving Domain Controller and Central Control Unit (CCU) Industry Report, 2024-2025
Autonomous Driving Domain Controller Research: One Board/One Chip Solution Will Have Profound Impacts on the Automotive Supply Chain
Three development stages of autonomous driving domain controller:...
Global and China Range Extended Electric Vehicle (REEV) and Plug-in Hybrid Electric Vehicle (PHEV) Research Report, 2024-2025
Research on REEV and PHEV: Head in the direction of high thermal efficiency and large batteries, and there is huge potential for REEVs to go overseas
In 2024, hybrid vehicles grew faster than batter...
Automotive AI Agent Product Development and Commercialization Research Report, 2024
Automotive AI Agent product development: How to enable “cockpit endorser” via foundation models?
According to OPEN AI’s taxonomy of AI (a total of 5 levels), AI Agent is at L3 in the AI development ...
China ADAS Redundant System Strategy Research Report, 2024
Redundant system strategy research: develop towards integrated redundant designADAS redundant system definition framework
For autonomous vehicles, safety is the primary premise. Only when ADAS is ful...
Smart Car OTA Industry Report, 2024-2025
Automotive OTA research: With the arrival of the national mandatory OTA standards, OEMs are accelerating their pace in compliance and full life cycle operations
The rising OTA installations facilitat...
End-to-end Autonomous Driving Industry Report, 2024-2025
End-to-end intelligent driving research: How Li Auto becomes a leader from an intelligent driving follower
There are two types of end-to-end autonomous driving: global (one-stage) and segmented (two-...
China Smart Door and Electric Tailgate Market Research Report, 2024
Smart door research: The market is worth nearly RMB50 billion in 2024, with diverse door opening technologies
This report analyzes and studies the installation, market size, competitive landsc...
Commercial Vehicle Intelligent Chassis Industry Report, 2024
Commercial vehicle intelligent chassis research: 20+ OEMs deploy chassis-by-wire, and electromechanical brake (EMB) policies are expected to be implemented in 2025-2026
The Commercial Vehicle Intell...
Automotive Smart Surface Industry Report, 2024
Research on automotive smart surface: "Plastic material + touch solution" has become mainstream, and sales of smart surface models soared by 105.1% year on year
In this report, smart surface refers t...
China Automotive Multimodal Interaction Development Research Report, 2024
Multimodal interaction research: AI foundation models deeply integrate into the cockpit, helping perceptual intelligence evolve into cognitive intelligence
China Automotive Multimodal Interaction Dev...